[ad_1]
Often considered neutral and impartial, algorithms in reality reproduce the inequalities of our society, or even worsen them. Mathilde Saliou, author of Technofeminism: How digital technology worsens inequalities, invites in-depth reflection on our way of creating algorithms. Interview.
The recruitment algorithmAmazon which favors men’s CVs ; the virtual assistant Siri who indicates where to find Viagra but not abortion centers ; black men wrongly accused by algorithms facial recognition of the American police… All these examples are the consequences of algorithmic bias. An algorithm is biased when its results are not neutral, fair, or even discriminatory.
In his book Technofeminism: How digital technology worsens inequalitiespublished in February 2023 by Grasset, journalist Mathilde Saliou demonstrates – among other things – that algorithms are not neutral, and depend who creates them, how, for what purpose and via what funding. Specializing in digital and equality issues for 10 years, she carried out this survey among multiple stakeholders in the sector in order to raise new questions about AI and potentially find new avenues for solutions. For Techniques de l’Ingénieur, she returns to the results of her investigation.
Engineering Techniques: How do algorithmic biases appear?
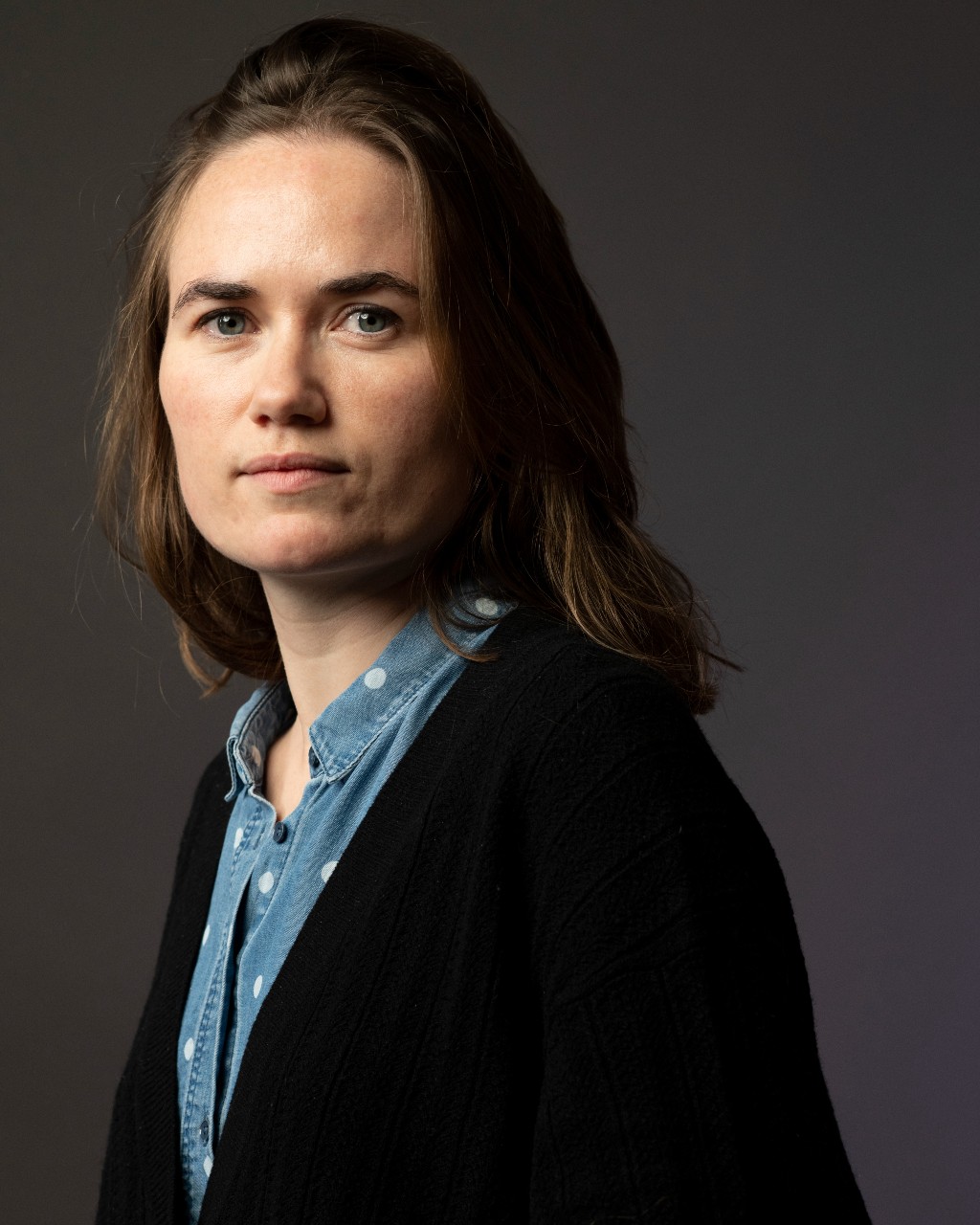
Mathilde Saliou: When we train an algorithm, we give it a dataset. However, this data can be biased from a statistical point of view if one type of data is over-represented compared to another. For example, if we ask an algorithm to recognize dogs and cats, and there were more photos of dogs in the training set, the algorithm will recognize dogs much more easily than cats. What could be trivial is no longer so when algorithms are used in society, with social data.
The problem is that our society is still unequal today, and these inequalities are reproduced in our algorithms. If the machine malfunctions, and for example recognizes white men better than black women, the latter will suffer inequalities linked to these errors. But when it comes to AI, we tend to think that the results are neutral and necessarily correct, so we rarely question the results.
In your book, you cite many examples of inequalities caused by algorithms. Can you give us some concrete examples?
In his study Gender Shades published in 2018, Joy Buolamwini, a researcher at MIT, analyzed the effectiveness of the facial recognition algorithms of the three most used models on the market at the time: IBM, Microsoft and Face++. She found that the algorithms recognized men better than women, and white people better than black people. So, when faced with a black woman, the algorithm had a good chance of making a mistake. The problem is that these dysfunctional technologies were already used in some countries and were used to process the video feed from surveillance cameras used by police stations. In the United States, I am aware of at least 6 cases of black people who were arrested because of an incorrect algorithmic result.
Another example, in 2021, the Dutch government resigned after a major administrative scandal in which thousands of families were wrongly accused of family allowance fraud. The government had deployed a fraud risk categorization algorithm which used sensitive data in terms of GDPR such as age, gender, linguistic abilities and, by extension, social and ethnic origins. Thus women, young people or people who spoke Dutch poorly found themselves more suspected of fraud and underwent numerous checks, sometimes several times in a row during the year. Aid could be suspended during these checks, which put people in very complex situations. This type of case has also taken place in Australia and in different states of the United States.
Finally, a more recent example in France, La Fondation des Femmes, the Femmes Ingénieures association and the NGO Global Witness took META to court in June 2023 for sexist discrimination of its algorithms. They found that the job advertisements broadcast on the network targeted populations in a stereotypical manner. Thus, offers for pilot positions were shown almost only to men, and those for childcare assistants, almost only to women. This mechanism places women in less well-paid positions, and contributes to perpetuating salary inequalities in society.
What are the causes of these biases?
One of the first causes comes from data. Sometimes, the datasets to train the algorithms have been poorly constructed, and for example use data from 40 years ago even though our society has evolved a lot since then. Sometimes, these datasets have been well constructed but reproduce the inequalities present in society.
Another cause comes from the people who create the algorithms. In the digital industry, 3 out of 4 people are men, and women often work in support positions such as HR, legal or communications. Thus, in Europe, only 16% of women participate in the construction of digital tools. However, these men, often white and well-off, will build tools according to their point of view and this vision will be very homogeneous within the team, which leads to oversights, blind spots, and biases.
Ultimately, it depends on which entity builds the tool and why. If it is a private company, its goal will mainly be to make money. Thus, the information prioritization algorithm at Facebook, whose economic model is based on advertising, will not be constructed in the same way as an entity like Wikipedia which promotes a model based on free access to knowledge. . In particular, we know that social media algorithms tend to push violent content rather than relevant content because this type of content creates more engagement. And that’s what they need to show more ads, and thus make more money.
Would more diversity in the digital world lead to fairer algorithms?
The lack of diversity, voluntary or not, prevents an awareness of the diversity of life experiences. This is a problem that I point out in digital technology but which is recurrent everywhere in society. However, tech often presents itself as neutral, impartial, and says that it produces universal tools, designed for everyone. But these white men who create it don’t know the lives of women, people from other social backgrounds, etc. By thinking of creating universal tools, they in fact reproduce their point of view and can create dysfunctional or even downright discriminatory tools. Thus, promoting diversity in the tech world would make it possible to multiply points of view, from the design of tools.
How can the practices of using data to train algorithms be problematic?
Not all practices are problematic, but I can cite two examples that raise questions. Since the launch of ChatGPT and Midjourney, many artists have carried copyright infringement complaint. Indeed, the algorithms used capture all the data they find online, without any framework, and have been able to train on thousands of works of art, without the consent of their authors. Another case in the United States, several American universities which were working on facial recognition have used video feed from campus surveillance cameraswithout anyone’s consent.
These examples raise many ethical questions, for example: do companies have the right to use all the data that Internet users put online? They are tempted to do so, because as it stands, the best algorithmic models need a very high number of data to function correctly. At the same time, many people are working on more ethical alternatives, with the creation of open source datasets or the implementation of models that require less data for equivalent results.
How can we create algorithms that are less, or even not, biased?
In addition to the need for greater diversity in the digital world, professionals in the sector should also be trained in social sciences, in order to better understand the mechanisms for reproducing inequalities. On the user side, it is necessary to disseminate digital culture more widely, to enable everyone to better handle the tools and develop critical thinking, without thinking that the result of a machine is necessarily better than human judgment. would have been.
Finally, we could act from a political point of view and put more frameworks on algorithm builders. On a European scale, the recently passed Digital Services Act is a start of reflection on the subject.
In your opinion, AI is not yet sufficiently supervised?
Certain issues such as the protection of privacy have existed for a long time, which gave rise to the GDPR in Europe and which already sets some limits. But now, we must ensure that the laws are applied, which requires real political will and therefore money injected into compliance verification.
Lots of laws and directives exist and already constitute a first base, but they have not really been designed for AI because its use is too recent. There are also many charters and directives, but they are non-binding and therefore not necessarily effective. I am also waiting to see what the regulation on artificial intelligence (AI Act), on which European legislators are working, will result.
In your book, you talk about a whole process of reflection and consultation that did not take place in society. Should we have more say in the use of AI?
Technology is an issue of power. Those who build and finance it do what they want and can shape as they wish the way in which users evolve in their digital creation. The question today is whether this power serves the common interest, or whether technologies only monopolize power, funds and opportunities in the hands of a few.
At the same time, many questions arise. Do we really want algorithms to govern every part of our lives? Should we create universal tools or would it not be better to seek greater efficiency by manufacturing specialized tools? All of these issues are very political and concern us all at a societal level.
Comments collected by Alexandra Vpierre
[ad_2]
Source link